A computational study on motion processing and learning in the brain, using support vector machines (SVM)
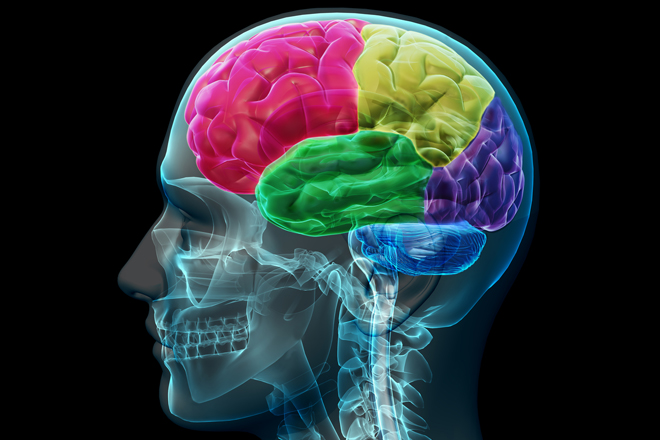
The middle temporal cortex (MT) is known to be a major brain area for processing visual motion information. A unique property of this area is the so-called motion oppenency. Namely, when there are opposite motion signals in the same retinal location, MT cells that otherwise respond vigorously to one of the motion directions will much reduce their firing, to the extent that the firing is on average the same as when responding to motion flicker noise (e.g., an empty TV channel). Why MT functions in this manner is little understood, although it is almost surely related to the well-known Motion Aftereffect (i.e., the perceived illusory upward motion after looking at a waterfall). In this regard, this motion opponency is likely important functionally, because there is evidence from our lab that motion perceptual learning could not reduce the strength of this oppenency (Thompson, Tjan, and Liu, 2013).
That perceptual learning study is on motion direction discrimination, whereby stimuli with opponent motions give rise to poorer discrimination performance than stimuli without opponent motions. Human participants nevertheless can train to improve their behavioral discrimination. The research question there is: is the behavioral improvement accompanied by reduced opponency at MT such that opponent motion stimuli could be better processed by MT cells, or motion opponency at MT is too important to alter, such that motion learning in the brain has to take place elsewhere, e.g., at V1?
While that study is experimental with both psychophysical and brain imaging techniques (fMRI), here we describe the companion study using computational techniques.
The first question we ask is this.
While MT is suppressed when opponent motion stimuli are presented, does MT still contain any motion directional information?
So far, the available data from physiology are from only a small number of MT cells (< 100). Although on average the cells respond similarly as to motion flicker noise, some cells nevertheless fire more than the average and some less. However, whether or not the cells that fire more than the average carry any motion directional information remains unclear. In fact, even if they don’t, it remains an open question whether there are any other cells in MT that respond to motion directions when MT is suppressed by the opponent stimuli.
Our approach is to use the best possible support vector machines (SVM), and to use our fMRI data from the entire MT, to try to pull out motion directional information. This will be compared with the discrimination performance by the same SVMs that use fMRI data from a control stimulus without opponent motions. The same comparison will also be carried out using fMRI data from V1, where no difference between opponent and non-opponent stimuli is expected.
We are very excited to wait and see whether MT can process any directional information under motion oppenency, because we will be the first in the world to find out. Meanwhile, we understand that, if the best SVMs cannot pull out any motion directional information, it does not mean that MT can absolutely not process any motion directional information (because there may be a better SVM that has not been discovered yet). Still, we will be able to know the upper bound of MT’s capacity, as compared to MT’s full capacity without motion opponency. Another cautionary note is that, even if directional information can be pulled out from suppressed MT, it doesn’t necessarily mean that down stream brain areas will use the information. In that case, it remains to be seen why directional information from MT is no longer useful while directional information is used all the time when MT is not suppressed. We will find this out and this is for sure worthy a publication.
The next question we ask is as follows:
After perceptual learning, can additional motion directional information be pulled out from MT’s fMRI data?
If yet, this indicates that learning takes place, at least in part, at MT that improves the quality of MT’s fMRI data. We will also correlate the amount of behavioral improvement with the amount of SVM improvement in directional discrimination. We will also look into other brain areas to compare pre- versus post-training, and to compare with the control stimuli that don’t exert motion opponency. This second question is also exciting, because we will have evidence, more direct than psychophysics, to tell us where motion perceptual learning takes place in the brain. Majed Samad, a graduate student is leading this exciting project above.
Thus far, we have talked about analyzing each brain area’s fMRI data separately and independently. In fact, these areas communicate with each other as a network.
Accordingly, our third question is as follows:
How brain areas as a network communicate with each other in terms of information flow to process motion directional information? How does perceptual learning change the pattern of the information flow?
This is cutting edge science. Our undergraduate student, Daniel Lin, will lead this project as his honors thesis. This project involves highly sophisticated computations, and Daniel is devoting his summer 2014 to learn the techniques at Stanford. This project is graduate level (and above) research, and surely has a great potential for publication. The UCLA Undergraduate Research Center is also excited about this research potential and has awarded Daniel the prestigious Undergraduate Research Scholars Program (URSP) scholarship to conduct this research in our lab. It will be an exciting year of new discoveries on the computational front.